If you’re in any kind of strategic role, you know the bliss of digging through your data and finding that one brilliant nugget of information that you can take action on. You get excited and start turning the gears to create the plan.
…Only to come across the fact that other tools or dashboards that should have identical information aren’t lining up. So you’re stuck not knowing what data to trust and disappointed as the excitement of the initial discovery fizzles out.
These inconsistencies are one of the biggest hurdles that leaders have to overcome, especially given the importance of data. In a survey by PwC of over 1,000 senior executives, organizations that are highly data-driven are 300% more likely to report better decision-making, in comparison to less data-driven organizations.
Given the reliance on data, these data discrepancies — or inconsistencies or differences found within data or between sets of data that should otherwise be identical — have the potential to severely impact the quality of your team or organization’s decision-making.
Common data discrepancies for SaaS teams
For SaaS teams, there are a few common ways that these data discrepancies may show up in your processes.
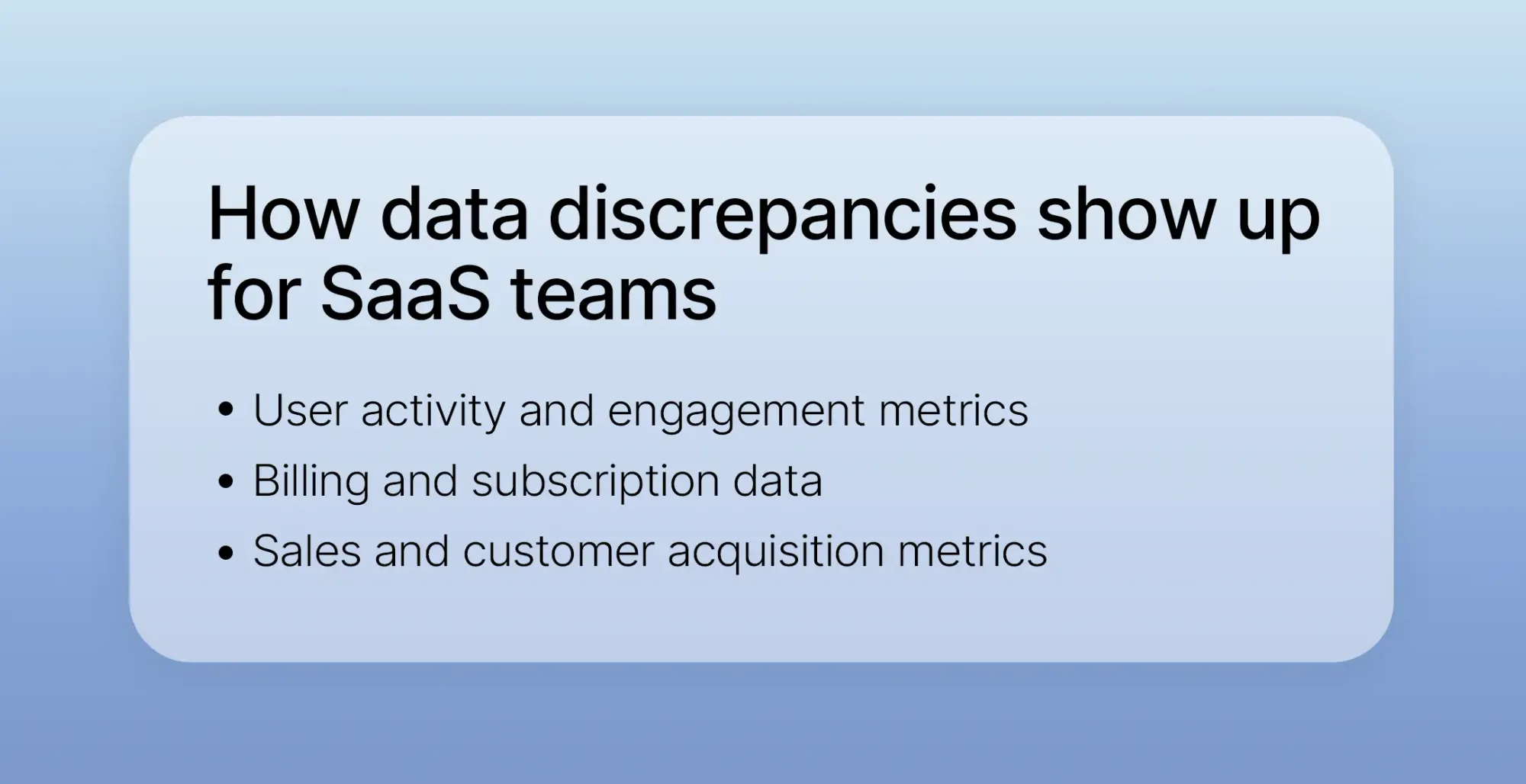
User activity and engagement metrics
For many SaaS teams, the data around how customers are using the platform and how much they’re using it is a treasure trove of ideas for creating a better user experience. By tracking metrics like time in the platform, feature adoption, drop-off rate, and more, your product team has more fuel to play up the platform’s strengths and get in front of any weaknesses.
Discrepancies that can sabotage the reliability of these engagement metrics can pop up, though.
For example, there may be a disconnect between the data reported by your platform’s internal analytics and external tools like Google Analytics. One may show significantly more user sessions or high rates of other engagement indicators than the other. This leads to conflicting interpretations of user behavior and product performance.
Billing and subscription data
Discrepancies can also show up in your billing and subscription data.
For example, a customer’s subscription status may show up as active in the CRM system but expired in the billing system due to synchronization issues. Not only can this harm financial reporting, but it can also lead to incorrect billing statements being sent to the customer, causing some confusion and likely dissatisfaction.
Sales and customer acquisition metrics
Understanding where your customers coming from is one of the best ways to shape your success going forward, so customer acquisition data is extremely valuable in making better strategic decisions.
This is another area where data discrepancy can rear its ugly head.
You may find that some of the sales data recorded in your CRM system doesn’t match up with the revenue figures that you grab from your financial software. It may show that your biggest revenue driver was an event campaign, when it reality it was paid social. This has the potential to lead you astray in allocating budget.
What makes these data discrepancies happen?
There are tons of potential culprits that are preventing your data from syncing across platforms. But here are the most common to look out for.
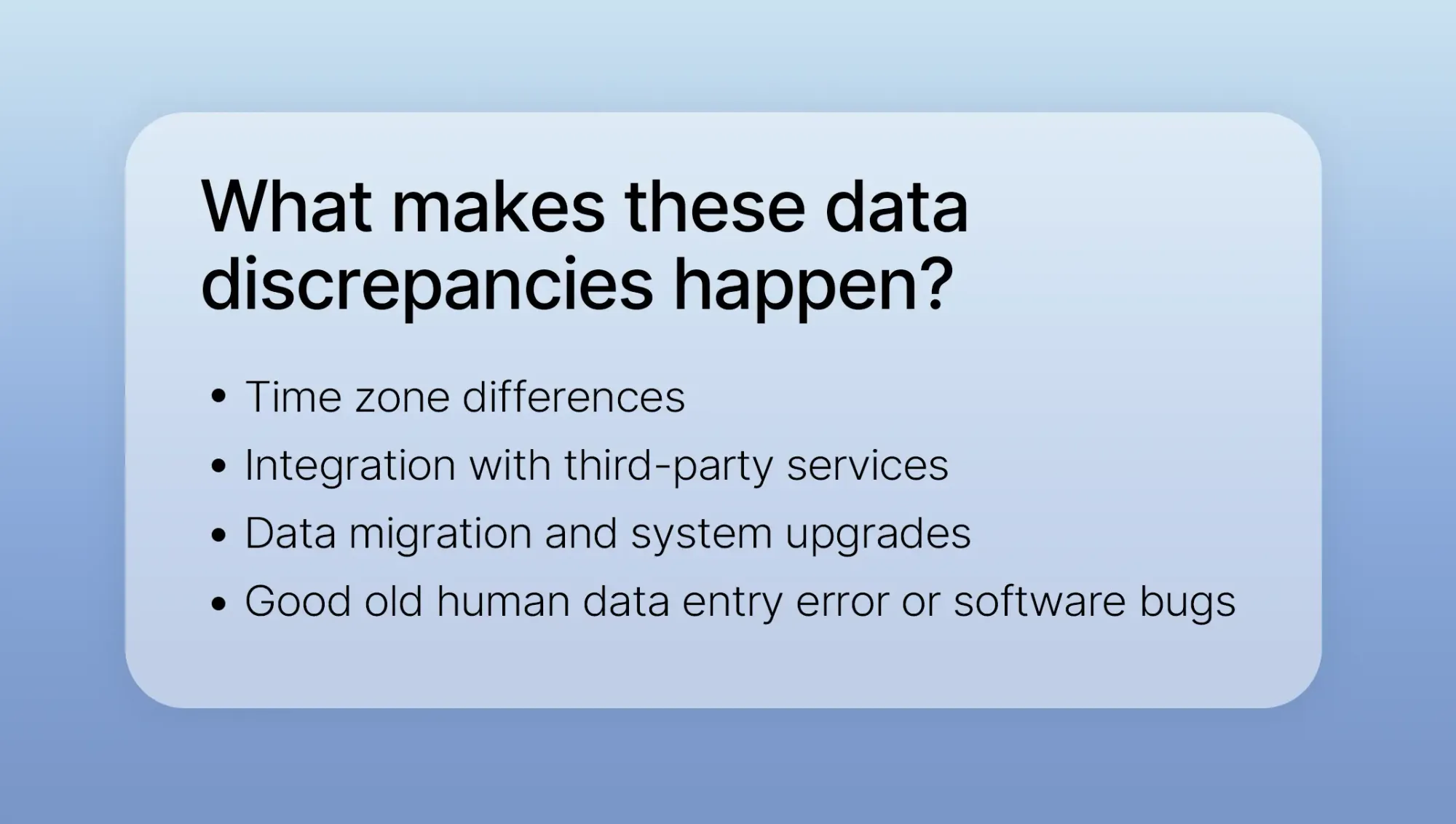
Time zone differences
Something as simple as a time zone may be at fault due to how the different platforms timestamp the data they collect.
Some platforms may timestamp data according to the local time zone of individual users, while others may timestamp it according to the time zone of the team member accessing the information, or the location of the headquarters.
These discrepancies may push certain interactions out of the monthly data collected on one platform but not another, for example.
Integration with third-party services
External integrations are essential for SaaS platforms to expand their reach and create a better, more well-rounded user experience. But these integrations can introduce some data discrepancies when APIs aren’t fully compatible or when there’s a sync error between systems.
For instance, a SaaS application that integrates an external email marketing tool might experience discrepancies if the tool sends back engagement data in a format not expected by the SaaS platform, leading to errors in how customer interactions are tracked and analyzed.
Data migration and system upgrades
Migrating data from one platform to another or performing system upgrades are necessary steps to keep SaaS platforms secure and efficient, but data migration tools does bring some risks to your data health.
There may be structural and format differences between the old and new system in a migration. This is especially common if you’re making the jump from a legacy system to a modern cloud-based system.
If thorough planning and testing aren’t done, you could potentially lose data or end up with misaligned records.
Good old human data entry error or software bugs
Sometimes, flukes happen, whether as a result of human error or bugs within a piece of software. These can be harder to anticipate because they’re more or less random, but they do have the ability to affect your data in both big and small ways. Here's why it's important to not only gather data but also protect it. There are many solutions for cloud storage and regular backups. One of the best is the AWS ecosystem, which offers various services to meet different business needs, such as air gap backups. AWS's scalability helps businesses implement AWS cost optimization strategies, ensuring they get the services they need while managing costs effectively.
You can’t just let these discrepancies slide
It may be tempting to shrug it off since you’re “not a data person.” Going back to our story in the beginning, you may be drawn to ignore the discrepancy and move forward anyway, essentially pretending you didn’t see it.
But this can lead to a slippery slope towards a sloppy and disorganized data culture. Nipping the problems in the bud is always the best strategy.
There are some tangible consequences to looking the other way, too.
First and foremost, it can sabotage the whole goal of being data-driven: making better, more informed decisions. As this author put it, “Ignoring and not removing bad data… is like blindly following a GPS without verifying its accuracy or knowing its end goal. You could potentially drive yourself into the ocean.”
And there are a variety of other consequences that will trickle in as a result of poor decision-making, including wasted money, time, and opportunities.
How to correct and prevent data discrepancies
Generally, dig deeper
Sometimes, data discrepancies may only seem elusive until you take the time to dig deeper. Hold the varying data up side-by-side and trace them to their source source. By following the path that the data took to get there, you can identify areas where things may have gone wrong.
Maybe it’s a time zone problem, a faulty API, or any of the other common culprits we mentioned before.
Improve data collection methods
Data issues often start at the collection stage, so making sure there are no breaks in the process can help you get more reliable data from the first step.
Take a step back and consider all of the different ways that your data is coming in and look for areas where errors may easily pop up.
One common strategy to create a better data collection process is to switch from manual data entry to automated data capture through methods like digital forms so that you can eliminate the possibility of human error.
Reinforce data integration techniques
You want to make sure that all systems and platforms in your stack are communicating effectively to minimize data discrepancies.
This starts with standardizing APIs to make sure that data being pushed and pulled between different systems maintains its integrity no matter how much it’s moving around.
You also don’t want to assume that data is a set-it-and-forget-it kind of thing. Schedule regular integrity checks to make sure that data across systems remains synchronized and accurate. You can even enlist automated testing tools that can simulate data transfers to predict potential breakdowns in the integration process before they occur in real life.
Even if you’re not the “data person” in charge of leading these initiatives, if you interact with data in a meaningful way, you still want insight into the standards put in place and strategy for keeping integrations running smoothly so that you can do your part to keep data clean.
Engaging with users
Let’s say you’re a product manager, a customer success agent, a salesperson, or a marketer who doesn’t have the know-how to dig into the data in a timely manner. Or even if you are a technical person who just can’t crack the case on why this data doesn’t line up.
Do you just move forward, ignoring the data discrepancy? No.
This is where you collect some real-time data. Dig into the anecdotal feedback you’ve gotten on recent surveys. Launch a new in-app survey to collect real-time data for users that you can use to validate which data point seems to be right.
You could also ask a handful of customers some questions face-to-face on your next check-in to try to get to the root of what the data is telling you.
As important as data is, your goal with the data is to make better decisions, and sometimes it takes an intentional effort to look up from the numbers and take in the full picture. Interactions with users can help to add some necessary color.